As businesses worldwide race toward digital transformation, one key lever is becoming increasingly indispensable: automation. Whether it’s reducing operational costs, enhancing accuracy, or boosting overall efficiency, automation has become a strategic pillar for future-ready enterprises. A recent McKinsey report found that 66% of businesses have already piloted automation solutions, while 31% have fully automated at least one function.
However, choosing the right kind of automation can feel overwhelming in the rapidly evolving tech landscape. Should your company invest in Robotic Process Automation (RPA) for quick wins? Leverage the power of Artificial Intelligence (AI) for deeper insights? Or embrace the next frontier—agentic AI, which brings a level of autonomy never seen before?
Each of these technologies has unique strengths and fits different stages of business maturity. RPA shines in handling high-volume, rules-based tasks with precision. AI adds intelligence to your workflows, learning from data and improving over time. And Agentic AI—emerging as a game-changer—can autonomously make decisions and adapt to real-time data, drastically reducing the need for human intervention.
To make an informed decision, it’s critical to understand their core differences, the real-world business problems they solve, and the return on investment they offer. Let’s break it down- so you can align the right automation strategy with your business goals.
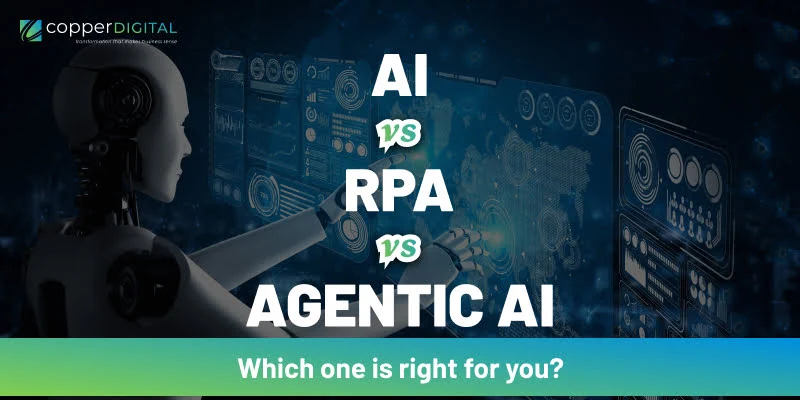
AI vs RPA vs Agentic AI
To understand the automation landscape, it’s important to distinguish between RPA, AI, and Agentic AI—not just in terms of what they do but in how they operate and the value they bring.
What is Robotic Process Automation (RPA)?
RPA is the entry point into automation for many businesses. It replicates human interactions with digital systems using bots that follow clearly defined rules. These bots can log into applications, extract data, enter information into forms, generate reports, and more. However, RPA is task-driven, not intelligence-driven. It performs tasks with speed and accuracy, but without understanding or learning from the data it handles.
A global bank drastically improved efficiency by automating its loan approval process, reducing processing times by 80%. The system used RPA bots to collect documents, verify data, and cross-check information, eliminating manual errors and accelerating customer approvals. This transformation not only enhanced customer satisfaction but also ensured compliance with regulatory requirements.
What is Artificial Intelligence (AI)?
AI Development goes beyond automation—it introduces cognitive capabilities. AI systems analyze structured and unstructured data, identify patterns, and make decisions based on algorithms and machine learning models. It continuously learns and improves with exposure to more data. This makes it invaluable for areas like customer analytics, predictive maintenance, and fraud detection.
In the healthcare sector, hospitals leverage AI-powered medical imaging to detect diseases earlier and more accurately. AI models analyze X-rays, MRIs, and CT scans to identify abnormalities that might be missed by the human eye, leading to faster diagnoses and improved patient outcomes. Hospitals can prioritize urgent cases and reduce misdiagnoses by integrating AI into diagnostic workflows.
Amazon uses AI to power its recommendation engine, which drives over 35% of its total revenue. Financial institutions use AI for real-time risk assessment and fraud prevention based on evolving behavioral patterns.
What is Agentic AI?
Agentic AI represents the future of automation. It combines the intelligence of AI with the autonomy of intelligent agents that can perceive their environment, set goals, and take actions independently. These systems are not just reactive; they’re proactive and adaptive. They interact with the environment in real time, learn from outcomes, and adjust their strategies to fulfill business goals with minimal or no human supervision.
Tesla’s Autopilot system is a prime example of Agentic AI in action. It continuously interprets environmental data from cameras, radar, and ultrasonic sensors to make real-time driving decisions.
Unlike rule-based automation, the system adapts dynamically to road conditions, pedestrian movements, and traffic patterns, enhancing both safety and driving efficiency.
Examples of Real-World Applications
Diving deeper into specific use cases, we see how automation reshapes various industries:
- RPA: Insurance companies automate claims processing, reducing turnaround times from days to minutes. RPA bots extract customer data, validate claim documents, and trigger approval workflows, minimizing human intervention and ensuring faster settlements. This improves both customer satisfaction and operational efficiency.
- AI: Airlines leverage AI to implement dynamic pricing models. By analyzing customer demand, competitor pricing, and booking trends, AI algorithms adjust ticket prices in real time, maximizing revenue and ensuring competitive pricing. This data-driven approach helps airlines optimize seat occupancy and boost profitability.
- Agentic AI: Autonomous drones are transforming warehouse management. These AI-powered drones scan inventory, detect discrepancies, and trigger automated replenishment requests—ensuring accurate stock levels and reducing manual labor. This level of autonomy is particularly valuable in large-scale logistics and e-commerce warehouses, where real-time decision-making is critical for efficiency.
Purpose
The purpose of each automation technology is aligned with the complexity of the tasks it handles and the level of intelligence it brings to decision-making.
- RPA (Robotic Process Automation) is designed to increase operational efficiency by automating structured, rule-based, and repetitive processes. It eliminates manual effort in transactional tasks, reducing errors and enhancing speed. This makes it an ideal solution for businesses that want cost savings and process consistency without overhauling their existing IT infrastructure. Common use cases of RPA include invoice processing, data entry, and report generation in departments like finance, HR, and customer service.
- The purpose of AI (Artificial Intelligence) is to augment decision-making by analyzing vast amounts of data, identifying trends, and generating predictive insights. Unlike RPA, which strictly follows programmed workflows, AI systems learn from patterns, improve over time, and can adapt to new data. This makes AI valuable for personalization, fraud detection, demand forecasting, and anomaly detection—helping businesses optimize processes beyond basic automation.
- Agentic AI operates with a higher level of autonomy and adaptability. It doesn’t just analyze data; it takes purposeful action toward business objectives while adjusting to dynamic environments. It goes beyond automation and prediction to self-correct, optimize workflows, and make strategic decisions without human intervention. This is particularly valuable in autonomous logistics, supply chain management, financial trading, and AI-driven customer interactions, where real-time responses and evolving decision-making are critical.
How It Works
The mechanics of each automation technology determine its level of intelligence, adaptability, and autonomy.
- RPA operates on a rules-based approach, executing predefined workflows without understanding the context. It interacts with applications the same way a human would—logging in, copying data, clicking buttons, moving files—but it strictly follows a script. While effective for repetitive tasks, it cannot analyze data or adapt to changing inputs unless manually reprogrammed.
- AI works through data-driven learning and pattern recognition. Using machine learning models (e.g., neural networks, NLP, decision trees), AI can extract insights, make predictions, and classify information based on historical and real-time data. Unlike RPA, AI requires continuous training with updated datasets to maintain accuracy. It’s commonly used for tasks like image recognition, chatbots, recommendation engines, and fraud detection, where decision-making requires learning from experience.
- Agentic AI is goal-driven and adaptive, leveraging real-time data, advanced neural networks, and reinforcement learning to make context-aware decisions. Unlike standard AI, which reacts to data inputs, Agentic AI proactively defines goals and adjusts its actions dynamically. It learns from both successes and failures, optimizing strategies on the fly. This allows it to function in high-stakes, unpredictable environments, such as self-driving vehicles, autonomous cybersecurity defense, and AI-driven financial markets.
Best For
Each automation technology is best suited for specific business challenges, depending on the complexity of tasks and the level of intelligence required.
- RPA (Robotic Process Automation) is ideal for businesses looking to automate structured, rule-based tasks in departments like finance, HR, and operations. It’s perfect for reducing manual workload, improving process speed, and minimizing errors without making significant changes to existing systems. Typical use cases include invoice processing, employee onboarding, data migration, and compliance reporting, where tasks follow a clear set of predefined rules.
- AI (Artificial Intelligence) is best suited for data-intensive decision-making, where analyzing large datasets can drive efficiency, accuracy, and business insights. AI helps businesses detect fraud in banking, predict equipment failures in manufacturing, and personalize customer experiences in marketing. It adds an intelligence layer to existing systems, enabling organizations to proactively solve problems rather than just automate tasks.
- Agentic AI is built for autonomous operations, where systems need to make real-time decisions, adapt dynamically, and complete tasks independently. It excels in complex environments like autonomous logistics, smart inventory management, real-time cybersecurity defense, and AI-powered customer engagement, where traditional automation falls short due to its lack of adaptability.
Use Cases
Each technology delivers unique value across industries:
Retail
- RPA: Automates stock level monitoring and invoice generation, ensuring seamless supply chain operations.
- AI: Analyzes customer behavior to drive personalized product recommendations and dynamic marketing campaigns.
- Agentic AI: Adjusts pricing dynamically based on competitor activity, demand surges, and market conditions in real time.
Healthcare
- RPA: Automates patient data entry, insurance claims processing, and appointment scheduling, reducing administrative burden.
- AI: Supports diagnostic accuracy by analyzing medical images, predicting disease risks, and assisting in treatment planning.
- Agentic AI: Powers autonomous patient triaging, emergency response systems, and AI-driven telehealth consultations.
Logistics & Supply Chain
- RPA: Automates shipment scheduling, inventory updates, and delivery confirmations, ensuring smooth logistics.
- AI: Optimizes route planning and demand forecasting by analyzing traffic patterns, weather conditions, and order history.
- Agentic AI: Enables autonomous drone delivery, robotic warehouse operations, and adaptive logistics networks that self-adjust in real time.
Need Type → Choose This
The right automation solution depends on your business needs:
- Need to eliminate manual, rules-based, repetitive tasks? → RPA is the fastest and most cost-effective option.
- Need intelligent systems that learn from data and improve decision-making? → AI provides the analytical power for continuous optimization.
- Need real-time, adaptive automation that operates independently? → Agentic AI enables self-sufficient, goal-driven systems.
Core Technology
The underlying technology powering each automation approach varies significantly:
- RPA is based on rule-based logic, screen scraping, and pre-configured scripts. It interacts with applications at the UI level, mimicking human actions without needing API integration or machine learning. While simple and highly effective, it is limited to structured environments with fixed processes.
- AI leverages machine learning models, deep learning, and statistical analysis. It processes vast datasets using supervised, unsupervised, and reinforcement learning algorithms to extract insights, detect patterns, and improve predictions. AI requires data pipelines and continuous training to maintain accuracy and relevance.
- Agentic AI integrates neural networks, agent-based modeling, reinforcement learning, and real-time sensor data. It operates autonomously by defining objectives, evaluating multiple options, and dynamically adjusting its behavior. This allows it to function in complex, real-time environments where decisions need to be made without human oversight.
Tools
Each automation technology is powered by specialized platforms designed to maximize efficiency and intelligence:
- RPA Tools: Platforms like UiPath, Automation Anywhere, and Blue Prism enable businesses to deploy low-code bots, automate repetitive tasks, and integrate seamlessly with enterprise software—all with minimal technical expertise.
- AI Tools: Frameworks like TensorFlow, PyTorch, IBM Watson, and OpenAI APIs empower businesses to train, deploy, and scale AI models for predictive analytics, natural language processing, and data-driven decision-making.
- Agentic AI Tools: Technologies such as LangChain, AutoGPT, and OpenAI Agents facilitate the creation of autonomous systems capable of reasoning, planning, and executing complex multi-step processes with memory and real-time adaptability.
Capabilities
Each technology excels in different aspects of automation:
- RPA: Delivers speed, accuracy, and consistency to routine workflows. It ensures strict rule enforcement, enhances compliance, and reduces manual workload in structured environments.
- AI: Brings cognitive intelligence—capable of pattern recognition, forecasting, and understanding unstructured data. It transforms raw data into actionable insights, making systems smarter and more adaptive.
- Agentic AI: Takes automation to the next level by introducing goal-directed behavior, contextual awareness, and self-correction. These systems can handle ambiguity, prioritize tasks, and replan dynamically.
Business Need
Automation technologies serve different strategic objectives based on an organization’s operational challenges and long-term vision.
RPA (Robotic Process Automation) is primarily focused on process optimization, making workflows faster, more efficient, and error-free. It’s ideal for businesses looking to reduce manual effort, eliminate repetitive tasks, and standardize operations in departments like finance, HR, and customer service. By automating structured workflows, such as invoice processing or employee onboarding, RPA frees up human employees to focus on higher-value tasks.
AI (Artificial Intelligence) is centered on decision support, providing businesses with advanced analytics, forecasting capabilities, and intelligent recommendations. AI-powered systems analyze historical data, detect patterns, and enable smarter business decisions. Industries such as banking use AI for fraud detection, while healthcare leverages it for predictive diagnostics. Organizations implementing AI must ensure proper data quality, governance, and infrastructure to maximize its benefits.
Agentic AI goes beyond task automation and decision support by enabling resilient innovation in dynamic environments. These systems act independently, continuously learning and adapting to changing conditions. For example, Agentic AI in logistics can autonomously reroute shipments based on real-time disruptions. This level of automation requires businesses to think beyond efficiency and embrace AI-driven transformation for long-term competitive advantage.
Ideal Fit
The adoption of automation depends on an organization’s digital maturity, technical capabilities, and strategic ambitions.
- RPA is best for organizations at the beginning of their automation journey. Companies with high volumes of rule-based, manual tasks but limited IT infrastructure can implement RPA quickly with minimal disruption. It works particularly well for businesses in finance, HR, and customer support, where structured workflows and repetitive transactions are common.
- AI is a natural fit for mid-to-large enterprises that already have data-driven strategies in place. These companies typically leverage AI to enhance decision-making, gain competitive insights, and automate cognitive tasks. AI adoption requires robust data management and investment in model training, making it well-suited for enterprises that want to unlock new efficiencies through machine learning and analytics.
- Agentic AI is ideal for innovation-driven enterprises that operate in fast-changing environments. These organizations seek to build autonomous, self-optimizing systems that can make decisions in real time without human intervention. Businesses in industries like advanced manufacturing, logistics, and aerospace benefit the most from Agentic AI, as they require intelligent automation capable of handling unpredictability and high operational complexity.
Industry Applications
Automation technologies create value across industries, but each type aligns with specific operational demands.
RPA: Used extensively in finance, healthcare, HR, and retail, where structured processes require efficiency and compliance. Banks automate loan approvals, hospitals streamline patient record updates, and HR departments manage payroll processing with RPA bots.
Also read: From Robotics to AI: The Role of Automation in Modern Healthcare
AI: Plays a critical role in e-commerce, telecom, insurance, and banking, where real-time data insights drive business decisions. AI personalizes shopping experiences, predicts customer churn in telecom, and automates insurance claims processing through machine learning.
Agentic AI: Gaining traction in automotive, logistics, manufacturing, and aerospace, where self-optimizing systems enhance productivity. For example, autonomous warehouse robots in logistics manage inventory dynamically, while AI-powered manufacturing systems optimize production lines based on demand fluctuations.
Decision-Making Approach
Automation technologies differ in how they process information and make decisions, impacting their flexibility and intelligence.
RPA: Follows a strict rule-based framework with zero contextual awareness. If a condition is met (e.g., “If invoice matches the purchase order, approve payment”), it executes the corresponding action. However, RPA lacks adaptability—if an exception occurs, human intervention is required.
AI: Uses data-driven decision-making to analyze historical trends and predict outcomes. AI models classify, forecast, and recommend actions based on statistical patterns. For example, AI in fraud detection scans financial transactions and flags suspicious activities based on risk scores, continuously improving with more data.
Agentic AI: Implements goal-directed reasoning, dynamically evaluating its environment and adjusting strategies in real time. Instead of following pre-set rules, it optimizes decisions based on long-term objectives. A self-driving car, for example, continuously reassesses road conditions, pedestrian movement, and vehicle speeds to determine the safest and most efficient route.
Intelligence
The level of intelligence in automation solutions ranges from rigid execution to proactive decision-making:
RPA: Lacks intelligence—it strictly follows pre-programmed scripts without learning or adapting. If a webpage layout changes or an unexpected data entry appears, the bot fails unless manually updated.
AI: Possesses reactive and predictive intelligence. AI can recognize patterns, anticipate trends, and make data-driven recommendations, but it typically operates within predefined parameters. For instance, AI in chatbots can understand user queries and respond, but it doesn’t act beyond its programmed scope.
Agentic AI: Embodies proactive and autonomous intelligence. It can set and pursue goals, plan multiple steps ahead, and even self-correct. In robotic process automation for supply chains, an Agentic AI system could reallocate resources, adapt to supply shortages, and modify logistics strategies without human intervention, ensuring seamless business operations even under unpredictable conditions.
Adaptability
Each automation solution varies in its ability to adjust to changing environments, workflows, or data inputs, impacting long-term scalability and maintenance efforts.
RPA: Has low adaptability and operates within rigid parameters. Any modifications to UI layouts, system updates, or process steps can break an RPA bot, requiring manual intervention and reprogramming. This makes it suitable for stable, repetitive tasks but challenging in dynamic environments where processes frequently evolve.
AI: Offers moderate adaptability as AI models can be retrained with new data to accommodate evolving requirements. For example, AI-driven fraud detection systems adjust to emerging fraud patterns over time. However, adaptation isn’t instant—it requires data updates, model fine-tuning, and human oversight to stay effective.
Agentic AI: Is highly adaptable and designed to evolve in real time. It continuously learns from new data, adjusts strategies, and adapts to unexpected changes without needing manual updates. This makes it ideal for autonomous decision-making in volatile environments, such as self-driving vehicles, cybersecurity threat detection, or intelligent supply chain logistics.
Input Type
The type of data an automation system can process determines its use case flexibility and the complexity of tasks it can handle.
RPA: Strictly works with structured data from sources like spreadsheets, CRM fields, or database tables. It cannot interpret unstructured inputs like emails, images, or spoken language, limiting its applications to transactional processes with clearly defined formats.
AI: Can process both structured and unstructured data, enabling it to analyze text, images, audio, logs, and sensor data. For example, AI can extract insights from customer reviews, classify emails, or detect anomalies in manufacturing equipment based on sound patterns.
Agentic AI: Handles all types of inputs, including real-time sensor feeds, live video analysis, behavioral tracking, and contextual data. It can process complex, multimodal inputs simultaneously, making it suitable for applications like predictive maintenance in factories, autonomous drones, or AI-powered personal assistants that adapt to user preferences in real time.
Learning Ability
The ability to learn and improve over time is a defining factor in how automation evolves from static execution to intelligent optimization.
RPA: Has no learning ability. It repeats predefined steps without any memory of past actions. If an input changes, the bot will fail unless explicitly reconfigured.
AI: Uses machine learning to improve over time. By analyzing historical data and feedback loops, AI can refine predictions, enhance classifications, and improve natural language understanding. However, it still requires human intervention for retraining and updating models.
Agentic AI: Uses reinforcement learning and memory-based reasoning to evolve autonomously. It continuously self-optimizes, improving performance based on real-world outcomes, environmental changes, and strategic goals. For example, an AI-powered trading system learns to adjust its investment strategies dynamically based on market fluctuations and risk factors.
Autonomy
The level of independence determines how much oversight an automation system requires to function effectively.
RPA: Has no autonomy—it executes tasks only when explicitly programmed and requires external triggers to start processes. If an unexpected scenario arises, it cannot adjust on its own.
AI: Provides partial autonomy—it can make decisions within defined boundaries but still needs human oversight. AI chatbots, for example, can handle customer queries but escalate complex cases to a human agent when necessary.
Agentic AI: Operates with full or near-full autonomy, executing goal-driven actions across multiple systems. It can self-correct, strategize, and make high-level decisions without constant human input, as seen in autonomous robotics, AI-driven cybersecurity defenses, and AI-powered fleet management.
Human Supervision
The degree of human involvement required varies based on how independently the automation system operates.
RPA: Needs frequent supervision, significantly when workflows change or unexpected inputs occur. Human intervention is required to fix errors, update scripts, and handle process exceptions.
AI: Requires moderate oversight to ensure model accuracy, retrain algorithms, and prevent biases or data drift. For example, AI-powered hiring tools must be monitored to avoid discriminatory biases in resume screening.
Agentic AI: Functions with minimal supervision, but ethical and compliance monitoring remain crucial. While Agentic AI can operate autonomously, human oversight is essential to ensure that decisions align with regulatory standards, ethical considerations, and organizational goals, especially in high-stakes environments like healthcare, finance, and national security.
Use Case Duration
The time required to realize value and ROI varies across RPA, AI, and Agentic AI, depending on implementation complexity, learning ability, and automation depth.
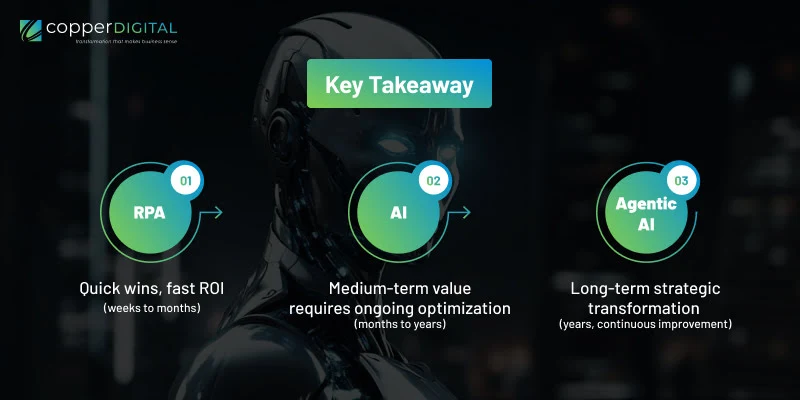
RPA: Best for short-term wins—it quickly deploys and delivers immediate ROI by automating repetitive, rule-based tasks. Since RPA operates without machine learning or complex integrations, businesses often see productivity gains within weeks or months. For example, automating invoice processing or employee onboarding can instantly reduce manual workload and operational costs.
AI: Provides medium to long-term value, involving data-driven insights, optimization, and smarter decision-making. AI implementations require data training, model fine-tuning, and iterative improvements before they reach peak efficiency. However, once operational, AI delivers scalable benefits, such as predictive maintenance in manufacturing, fraud detection in banking, or personalized recommendations in e-commerce, that compound over time.
Agentic AI: Designed for long-term transformation by enabling autonomous systems that continuously learn, self-optimize, and generate increasing returns. Unlike RPA, which delivers immediate efficiency, Agentic AI builds strategic advantages over time by adapting to evolving environments. Examples include self-driving logistics fleets, autonomous investment management, or AI-powered cybersecurity systems that become more effective the longer they operate.
Key Takeaway:
- RPA = Quick wins, fast ROI (weeks to months)
- AI = Medium-term value, requires ongoing optimization (months to years)
- Agentic AI = Long-term strategic transformation (years, continuous improvement)
Organizations should align their automation investments with their business goals—RPA for immediate efficiency, AI for strategic insights, and Agentic AI for long-term competitive advantage.
Enterprise Complexity
Implementing automation technology requires different levels of organizational readiness, infrastructure maturity, and cross-functional collaboration.
RPA is generally low in complexity and ideal for businesses looking to automate straightforward, repetitive processes. Since it mimics human interactions with software applications, RPA can often be deployed with minimal changes to existing systems. It is best suited for departments like finance or HR, where structured tasks dominate.
AI brings a moderate level of complexity. Deploying AI requires quality data pipelines, proper infrastructure, and dedicated teams to train, monitor, and optimize models. Organizations must also factor in data governance, algorithm transparency, and user adoption. This makes AI more suitable for mid-to-large enterprises with existing digital maturity.
Agentic AI, on the other hand, introduces a high degree of enterprise complexity. It requires not just robust data environments and integrated systems but also governance mechanisms to manage autonomy and ethical use. For instance, deploying Agentic AI in autonomous logistics or decision-making systems often involves real-time sensors, continuous learning loops, and compliance oversight, demanding both technical and strategic investment from leadership.
ROI Timeline
Understanding when to expect returns from your automation investments is critical to justifying and sustaining transformation initiatives.
RPA offers the fastest return on investment. Since it automates well-defined manual tasks, such as data entry or payroll, it starts delivering value within weeks or months. Businesses often see immediate cost savings and productivity gains.
AI takes longer but pays off with deeper insights and long-term optimization. It typically requires 6 to 18 months to build, train, and deploy models that start providing measurable benefits, such as fraud detection in financial services or demand forecasting in retail.
Agentic AI has a longer ROI horizon due to its complexity and cost of deployment. However, its autonomous capabilities result in compounding returns over time. For example, self-adjusting supply chain systems using Agentic AI can reduce human intervention and error, saving millions annually in enterprise environments.
Limitations / Challenges
Each automation technology presents specific hurdles that must be addressed to ensure a successful implementation.
RPA is limited to structured environments. It struggles with processes that involve dynamic inputs or decision-making. If the underlying interface or workflow changes, the bots often break, requiring reconfiguration.
AI needs access to large volumes of high-quality, well-labeled data to perform optimally. Additionally, training models are computationally intensive, and the output may sometimes reflect biases present in the data, posing ethical and operational risks.
Agentic AI brings complexity to a new level. It demands significant upfront investment in infrastructure, skilled talent, and ethical frameworks. The autonomy it offers also introduces governance challenges, especially when operating in regulated industries like healthcare or finance.
Cost & Implementation Effort
Cost is often a deciding factor in choosing the right automation solution, but it’s essential to weigh it against long-term benefits.
RPA is the most budget-friendly option to get started with automation. Its implementation usually involves low capital expenditure and minimal disruption to existing systems, making it ideal for quick deployment.
AI demands a medium investment. It requires building or accessing a data infrastructure, hiring data scientists or ML engineers, and continuously training and tuning models. However, its long-term value justifies the setup cost in many cases.
Agentic AI has the highest implementation effort. It involves complex integrations with live data systems, regulatory audits, and AI governance policies. Only organizations with strategic automation goals and high innovation tolerance should consider this route.
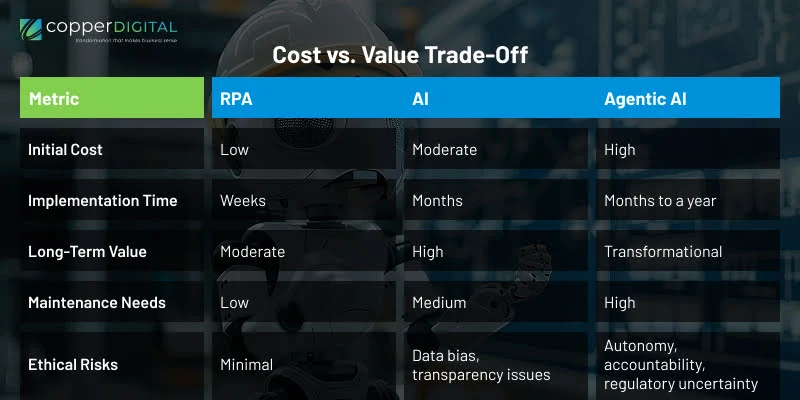
Risk Factors
Every automation tool introduces certain risks that enterprises must manage through proper oversight and planning.
RPA is sensitive to any changes in user interfaces or process logic. A slight UI update can halt bot operations, affecting business continuity. Moreover, since it lacks contextual understanding, it can’t handle exceptions well.
AI presents challenges around data privacy and algorithmic transparency. If not properly governed, AI models can inherit biases from training data and make decisions that are hard to explain—a growing concern with compliance and ethics teams.
Agentic AI amplifies both opportunity and risk. The potential loss of human oversight introduces concerns about accountability and liability, especially when systems act independently. Industries must also grapple with evolving regulations around autonomous decision-making and AI ethics.
Integration Complexity
Implementing automation solutions is not just about the tool—it’s about how seamlessly it fits into your existing tech stack.
RPA offers the simplest integration path. It sits on top of current systems, mimicking human actions without requiring backend access or major architectural changes. This makes it ideal for companies relying on legacy software or those that want fast deployment with minimal disruption.
AI demands a more robust and cleaner data infrastructure. Integration often involves connecting various data sources, APIs, and sometimes third-party analytics platforms. Successful AI integration also requires coordination between IT, data science, and business teams to ensure the outputs are relevant and usable.
Agentic AI introduces a high level of integration complexity. It typically involves real-time data ingestion from multiple systems (e.g., sensors, ERPs, CRMs), seamless interoperability across functions, and feedback loops for learning. Integration isn’t just technical—it also includes ethical frameworks, cross-departmental orchestration, and governance mechanisms.
Best Adoption Strategy
The path to automation success lies in how strategically you adopt these technologies.
RPA should start small. Automate a few well-defined, high-volume tasks in departments like finance or HR to show immediate ROI. Then scale based on performance and team comfort.
AI adoption benefits from piloting high-impact use cases, such as fraud detection, predictive maintenance, or customer segmentation. Begin with areas where clean data is available and the impact of better insights can be measured.
Agentic AI requires long-term planning. It’s not just about automating tasks—it’s about redesigning workflows to enable autonomy. Adoption should start with defining business goals, establishing AI ethics guidelines, and setting up compliance oversight before selecting pilot domains like logistics or customer experience.
Scalability Potential
Scalability determines how well your automation investment grows with your business.
RPA has limited scalability. It works well when task complexity is low and processes are static. But it struggles with scale when exceptions increase or when processes change frequently, requiring constant bot reconfiguration.
AI is highly scalable across functions. Once data infrastructure and models are in place, AI can be extended to multiple departments—marketing, sales, finance, operations—delivering exponential returns as models are reused or fine-tuned.
Agentic AI is exceptionally scalable, especially in systems that benefit from autonomy. From self-optimizing supply chains to real-time customer service bots, Agentic AI can manage a wide range of complex scenarios without human input, compounding returns over time.
Ethical & Compliance Considerations
Automation doesn’t just change workflows—it raises new ethical and legal questions.
RPA has minimal ethical concerns. Since it follows deterministic rules, it’s easy to audit and govern. However, over-reliance on RPA without rethinking processes can create bottlenecks elsewhere.
AI introduces ethical risks related to bias, data privacy, and explainability. Compliance with data regulations like GDPR or CCPA becomes critical. Businesses must ensure transparency in how models make decisions and safeguard personal information.
Agentic AI demands a deeper ethical framework. These systems can act independently, raising concerns about fairness, accountability, and control. From autonomous vehicles to financial bots, organizations must ensure that Agentic AI behaves in a safe, transparent, and non-discriminatory way, while complying with emerging AI governance laws globally.
Maintenance & Upkeep
Automation isn’t set-and-forget—it needs to be maintained to stay effective.
RPA requires low maintenance as long as the underlying systems remain unchanged. However, any UI change or process update can disrupt bot functions, requiring manual reconfiguration.
AI needs regular updates. Models must be retrained on new data to stay relevant. It also requires performance monitoring to detect model drift and avoid decision-making based on outdated insights.
Agentic AI involves ongoing system auditing, reinforcement learning updates, and ethical oversight. These systems continuously evolve, which means regular checks are needed to ensure alignment with business goals and regulatory compliance.
Competitive Advantage
Automation is not just about efficiency—it’s about building a strategic edge.
RPA enhances operational efficiency by reducing errors, speeding up processes, and freeing up human capital for higher-value tasks.
AI creates smarter organizations. With predictive and prescriptive insights, businesses can make faster, more informed decisions, respond to market trends, and better serve customers.
Agentic AI unlocks a future-facing competitive advantage. It enables real-time, autonomous innovation, allowing businesses to move from being reactive to self-optimizing entities that anticipate needs, reduce waste, and lead their industry with agility.
Want a quick comparison? Download our RPA vs. AI vs. Agentic AI cheatsheet for a concise breakdown of their differences and ideal use cases!
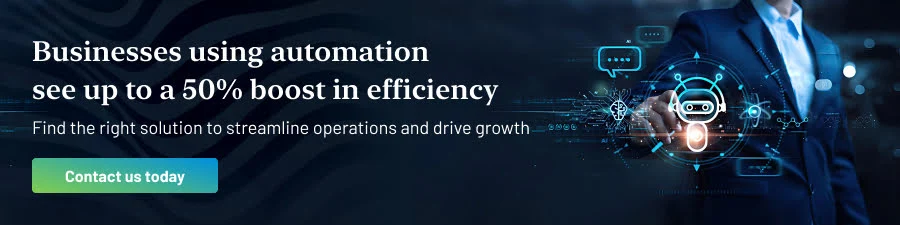
Final Thought: Which One Is Right for You?
If you’re looking to dip your toes into automation, RPA provides the quickest, most affordable route. For smarter decision-making and insights, AI unlocks vast potential with predictive and cognitive capabilities. But if you’re aiming to build the enterprise of the future—capable of thinking, reacting, and optimizing on its own—Agentic AI offers a transformative leap.
At Copper Digital, we specialize in guiding businesses across all three—from RPA deployment to AI solutions and Agentic AI integration. Let’s explore what intelligent automation can do for your operations. Contact us today to begin your journey.