The healthcare industry is on the cusp of a technological revolution with the potential to transform how we deliver patient care. And at the forefront of this revolution is the groundbreaking implementation of Machine Learning in Healthcare!
Machine learning, a subset of artificial intelligence, equips computers to learn and improve from experience without relying on explicit human programming. By processing massive amounts of healthcare data, machine learning algorithms can identify patterns, make predictions, and unearth valuable insights to help providers deliver superior patient outcomes and elevate overall healthcare services.
The potential of machine learning in healthcare is enormous. From diagnosing diseases to predicting the likelihood of future health issues, this technology is already being used to revolutionize how healthcare is delivered. For example, machine learning algorithms can analyze medical images with accuracy and speed that exceeds human capabilities, leading to a more accurate and efficient diagnosis of diseases such as cancer.
Machine learning also personalizes treatments to an individual’s unique genetic makeup, providing more targeted and effective therapies. With the help of machine learning, doctors can identify specific genetic mutations that may predispose a patient to certain diseases, enabling them to take preventative measures and provide early interventions.
But the applications of machine learning in healthcare are broader than individual patient care. It can also be used to identify public health trends and predict the likelihood of future epidemics. By analyzing massive amounts of data from sources such as social media and online search patterns, machine learning can detect early warning signs of potential health crises, enabling healthcare providers and policymakers to take proactive measures to prevent the spread of diseases.
In this blog, we will dive into the transformative power of machine learning in healthcare, exploring its current applications, potential future developments, and the ethical and privacy considerations of using this technology. Join us as we explore the exciting potential of machine learning to revolutionize healthcare and improve patient outcomes.
Also, Read – The Influence of Artificial Intelligence in Healthcare
Applications of Machine Learning in Healthcare: An Overview
Machine learning is revolutionizing the healthcare industry by providing numerous benefits, from maximizing hospital efficiency to an accurate diagnosis. Here are some of the significant applications of machine learning in healthcare that are transforming the industry and enhancing patient outcomes.
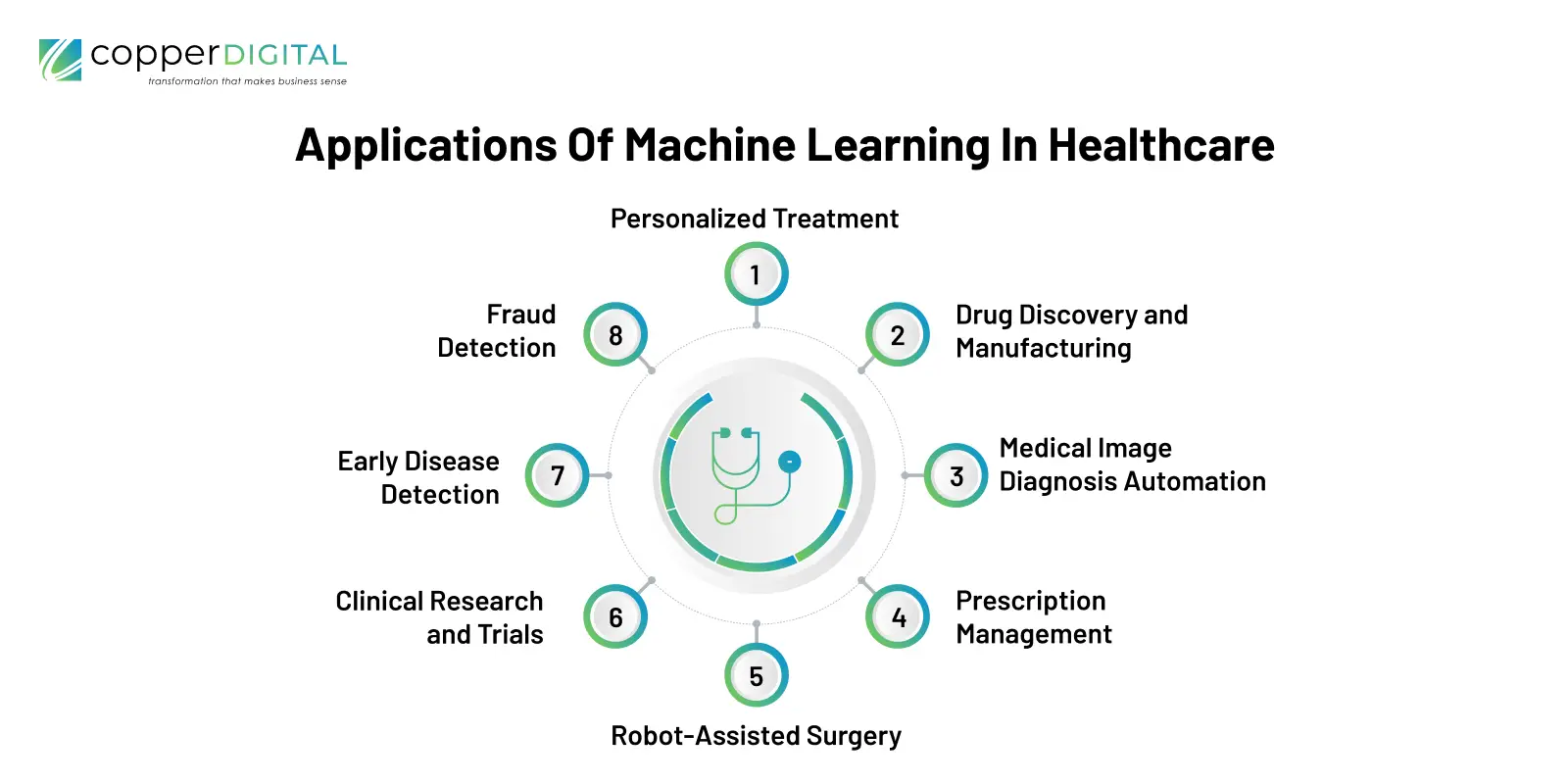
- Personalized Treatment
Implementing machine learning in healthcare has yielded remarkable advancements, with one of the most notable use cases being personalized treatment. Machine learning algorithms empower healthcare providers to deliver tailored and precise care to each individual through meticulous analysis of a patient’s medical history, symptoms, and test results. This revolutionary approach enables doctors to craft bespoke treatment plans and prescribe medication targeting the patient’s unique disease.
By leveraging electronic health records, healthcare professionals can swiftly determine the optimal course of action, resulting in faster and more effective treatment. Furthermore, machine learning aids doctors in assessing a patient’s readiness for medication changes, thus ensuring that the correct treatment is administered from the outset.
- Fraud Detection
The fight against fraudulent activities in the healthcare industry has become a paramount concern, given that a startling 3% of healthcare claims in the US are found to be fraudulent, resulting in an annual loss of an astounding one hundred billion dollars. Thankfully, modern machine learning models have become a powerful tool to combat this menace.
By leveraging advanced ML algorithms, healthcare providers can now effectively identify and thwart invalid claims before they are processed while simultaneously expediting the approval, processing, and payment of legitimate ones. In addition to curbing insurance fraud, these models safeguard sensitive patient data. Prominent healthcare institutions, such as Harvard Pilgrim Health, are leading the charge in adopting innovative AI and ML-based fraud detection systems, enabling them to scrutinize claims and easily spot suspicious behavior.
- Early Disease Detection
The timely detection of diseases is paramount to implementing effective treatment plans and ensuring optimal patient outcomes. Leveraging the power of cutting-edge machine learning techniques, a synergistic approach involving supervised and unsupervised algorithms offers unparalleled support to healthcare providers in the early diagnosis of ailments. In addition, employing sophisticated algorithms to compare new patient data with historical disease profiles enables medical practitioners to quickly identify potential warning signs, take proactive measures, and provide timely interventions that can significantly improve patient prognosis.
- Clinical Research and Trials
Aiding in clinical research and trials is a vital task that entails significant investments of time and resources. Ensuring the safety of novel drugs and medical interventions necessitates a rigorous and meticulous approach to the research process. Nonetheless, there are situations where expediency is paramount, as in the case of the urgent need for effective COVID-19 vaccines.
Machine learning algorithms have revolutionized the clinical research landscape, enabling streamlined and accelerated processes. These cutting-edge algorithms facilitate the selection of optimal trial samples, allow the collection of more comprehensive and relevant data points, facilitate ongoing data analysis from trial participants, and minimize errors arising from data analysis.
- Robot-assisted Surgery
The advent of robot-assisted surgery, fueled by machine learning, has ignited a paradigm shift in the field of surgery, delivering unparalleled levels of precision and practicality. These state-of-the-art systems execute intricate procedures with a remarkable reduction in blood loss, side effects, and pain while simultaneously facilitating faster and more comfortable post-operative recovery.
One illustrious case in point is the Maastricht University Medical Center, which has harnessed the potential of an ML-powered surgical robot to perform intricate suturing of tiny blood vessels, scarcely exceeding 0.03 millimeters in width. The use of machine learning in medicine and healthcare has empowered professionals and surgeons with instantaneous access to real-time data and invaluable insights concerning their patients’ current health status, facilitating informed decision-making before, during, and after surgical interventions, ultimately leading to superior clinical outcomes.
- Prescription Management
The gravity of prescription errors in the US cannot be overstated, with an annual death toll of 5,000 to 7,000 people attributed to these errors. These lapses often result from poor electronic health record (EHR) interfaces that cause physicians to select incorrect medications or make errors in dosing units. However, there is hope in the form of Machine Learning (ML) technologies that can play a vital role in averting such tragedies.
ML models can analyze and compare new prescriptions to established patterns by leveraging historical EHR data. Any deviations from the norm are immediately flagged, empowering doctors to review and adjust the medication to prevent harmful consequences.
To illustrate, Brigham and Women’s Hospital deployed an ML-powered system to identify prescription errors, and the results were staggering. The system flagged over 10,000 potential mistakes in a year, with 79% deemed clinically valuable, leading to $1.3 million in healthcare-related cost savings.
Furthermore, an ML-based error detection system saves money and enhances the quality of care by preventing drug overdoses and related health risks. With the potential to save countless lives, adopting ML in healthcare is a promising development that cannot be ignored.
- Medical Image Diagnosis Automation
Revolutionizing medical diagnosis through advanced technology, hospitals, and clinics are implementing state-of-the-art machine learning algorithms to automate the recognition of abnormalities in various medical images, ranging from MRI to radiology scans. By harnessing the power of image recognition, medical professionals can now expedite the diagnosis of conditions such as liver and kidney infections and tumors and even enhance cancer prognosis.
One of the most groundbreaking examples of this technology is the research conducted by Stanford University. Researchers developed an algorithm to accurately diagnose skin cancer using more than 130,000 clinical images dataset. The algorithm outperformed dermatologists in identifying melanoma, the deadliest form of skin cancer, and could potentially be used to improve early detection and treatment of this condition. In addition, this technology can improve patient outcomes and reduce healthcare costs associated with skin cancer diagnosis and treatment.
- Drug Discovery and Manufacturing
The remarkable advancements have revolutionized drug discovery and creation in machine learning, which is regarded as a crucial asset in healthcare. With its unparalleled ability to identify new drugs, ML offers a tremendous advantage to the pharmaceutical industry, hospitals, and patients, opening up new treatment avenues and driving economic growth. Furthermore, using ML in drug creation has significantly expedited the process, making it much more cost-effective.
Atomwise, a pioneering pharmaceutical company, leverages the power of supercomputers to extract therapies from molecular structure databases. Using its cutting-edge deep learning technology, Atomwise was able to redesign existing drugs to treat the Ebola virus in 2015, a feat that would have taken years through traditional methods. In just one day, Atomwise’s ML-based technology successfully identified two drugs that could help mitigate the epidemic’s risk, demonstrating ML’s incredible potential in drug discovery and creation.
After exploring the primary applications and use cases of machine learning in healthcare, it is time to delve into the obstacles associated with incorporating ML technologies pertinent to this industry.
So, let’s get started!
Also, Read – MACHINE LEARNING FOR DUMMIES 2023
Obstacles to Implementing Machine Learning in Healthcare
Adopting advanced technologies such as AI and ML in healthcare is challenging. The industry faces numerous obstacles, including the scarcity of high-quality data and concerns regarding patient safety, when leveraging ML-powered software and tools. Let us examine these challenges in further detail:

Regulatory Barriers: The highly regulated environment surrounding healthcare can present significant challenges when implementing ML technologies. Organizations may need help navigating the complex and time-consuming compliance process required for ML implementation. This can delay progress and hinder the adoption of technologies that can improve patient outcomes and drive economic growth.
Data Quality and Privacy Concerns: The success of ML algorithms is highly dependent on the quality and completeness of healthcare data. Unfortunately, healthcare data is often fragmented, prone to errors, and incomplete, which can undermine the reliability of ML models. Furthermore, privacy concerns related to patient data can limit the data used for training these models.
Resistance to Change: Healthcare is a traditional industry, and stakeholders may resist implementing new technologies due to a fear of the unknown or perceived job insecurity. Effective communication and collaboration between stakeholders are essential to overcome this resistance and to promote the adoption of ML technologies that could benefit patients.
Integration with Existing Systems: Integrating ML into existing healthcare systems can be challenging, especially if the systems need to be updated or designed to handle large amounts of data. A robust infrastructure that can accommodate ML algorithms and ensure seamless data exchange between different systems is essential for effective integration.
Lack of Standardization: The lack of standardization in data formats and medical terminologies presents a significant obstacle to the widespread implementation of ML in healthcare. With standardized data, creating accurate and reliable ML models can be easy. Standardizing data formats and medical terminologies is crucial for realizing the full potential of ML in healthcare.
Ethical Considerations: The use of ML in healthcare raises ethical concerns regarding bias, transparency, and fairness. There is a risk that ML algorithms may perpetuate existing biases in healthcare, leading to unfair or unequal treatment of patients. Ensuring that ML algorithms are transparent, unbiased, and fair is crucial for building trust and promoting equity in healthcare.
Cost and Resource Constraints: Implementing ML in healthcare requires significant time, money, and resource investment. This can be a barrier for many healthcare providers, especially those in resource-limited settings. However, the potential benefits of implementing ML in healthcare outweigh the initial investment costs.
Despite these challenges, the potential benefits of implementing machine learning in healthcare are vast, including improved patient outcomes, reduced costs, and increased efficiency.
Machine learning algorithms can analyze vast amounts of data to identify patterns and predict outcomes, enabling healthcare providers to make more informed decisions about patient care. However, implementing machine learning in healthcare requires addressing several challenges, such as ensuring data quality and privacy, addressing ethical concerns, and building trust among patients and healthcare providers.
Addressing these challenges will require collaboration between stakeholders, including policymakers, healthcare providers, and technology companies, to realize the full potential of machine learning in healthcare.
Also, Read – Top 7 Machine Learning Trends to Look Out for in 2023-24
The Future of Machine Learning in Healthcare
Machine learning (ML) is transforming healthcare as we know it, with endless possibilities to improve patient outcomes, clinical practice, and pharmaceutical operations. Yet, despite some challenges, according to Grand View Research, the market for AI and ML in healthcare is projected to experience an astounding compound annual growth rate (CAGR) of 38.4% between 2022 and 2030.
The increasing availability of patient health data, the demand for personalized medicine, and the need to reduce healthcare costs are the major driving forces for this growth. With the emergence of programmed robots assisting doctors during surgeries and ML-powered technologies helping them minimize the risks of complex treatments, the future of healthcare looks more promising than ever.
Moreover, ML is advancing the field of radionics and enabling “virtual biopsies” for more accurate and faster insights, allowing healthcare providers to make better decisions. Early warnings for conditions such as seizures or sepsis, which require intensive analysis of complex datasets, are also being made possible by ML-powered tools. Leveraging ML for risk scoring, clinical decision support, and early alerting are some of the significant areas of development for this groundbreaking approach.
As the use of ML in healthcare continues to expand, it is crucial for healthcare software development companies, along with other stakeholders like policymakers and healthcare providers, to collaborate and leverage this technology to drive better patient outcomes, reduce costs, and increase efficiency.
The healthcare industry must adopt ML technology and harness its potential benefits to ensure a healthier and more prosperous future. Healthcare professionals’ and clinicians’ active utilization of innovative ML technologies is essential for maximizing these benefits. Healthcare software development companies have a pivotal role in this adoption process by creating and implementing ML-based solutions that tackle industry challenges. Thus, stakeholders should collaborate to embrace this transformative technology for enhanced patient outcomes, decreased costs, and improved healthcare efficiency.
Take advantage of the opportunity to revolutionize healthcare through ML technology! Instead, connect with healthcare software development companies to learn more about implementing ML-based solutions and stay ahead of the curve.