Home health care agencies today face mounting pressures – from staff shortages and caregiver burnout to rising hospital readmissions and delayed care for patients at home. Recent data shows that 89% of home care providers have had to deny new patients due to workforce shortages, with small agencies turning away over 500 hours of care monthly. This gap leaves vulnerable seniors without timely support and contributes to avoidable complications. The good news is that technology is catching up to these challenges. Predictive AI in home health care offers a promising path to preventive health care with AI, enabling providers to anticipate risks, allocate resources efficiently, and keep patients healthier at home. This comprehensive guide explores how AI-driven predictive analytics and remote monitoring are transforming home health, with real-world examples, stakeholder benefits, an implementation roadmap, future trends, and expert insights. By embracing these tools as part of a broader digital transformation in home health care, agency owners and tech leaders can improve outcomes, enhance home health efficiency with AI, and gain a competitive edge. Let’s dive in.
Core Challenges in Home Health Care
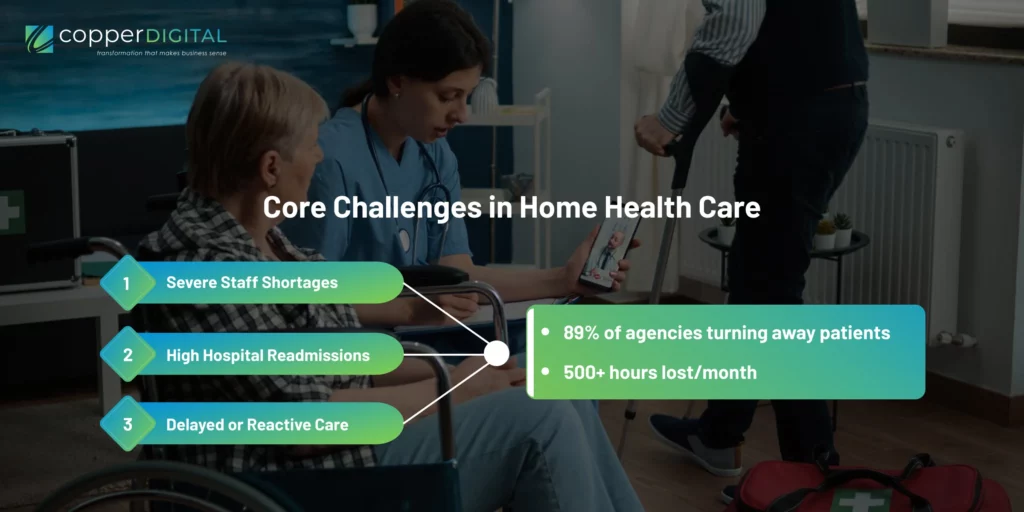
Home health organizations serve a rapidly growing aging population, but they grapple with serious systemic problems that threaten care quality and business viability:
- Severe Staff Shortages: Demand for in-home care is surging as 88% of seniors wish to age in place, yet the supply of nurses and aides can’t keep up. The direct care workforce is expected to add over 1 million new jobs by 2031, but filling these roles is proving difficult. This workforce crisis forces agencies to turn away patients or delay services, leading to longer wait times and overworked staff. One study found that nearly 9 in 10 home care agencies had to refuse patients due to a lack of staff – a situation that puts seniors at risk of unmet needs and increases caregiver burnout.
- High Hospital Readmissions: Patients discharged to home care often end up back in the hospital due to complications or inadequate follow-up. Preventable readmissions drive up costs and indicate lapses in timely intervention. For example, without proactive monitoring, a heart failure patient’s weight gain or a diabetic senior’s fluctuating blood sugar might go unnoticed until a crisis occurs. Reducing these readmissions is critical – not only to avoid Medicare penalties but to improve patient outcomes and satisfaction. Traditional approaches like periodic check-ins often react after a condition worsens rather than predicting issues ahead of time.
- Delayed or Reactive Care: In many home health settings, care is provided on a schedule or in response to obvious symptoms. This reactive model can result in missed early warning signs. Delayed care means a minor issue (like slight swelling or subtle behavior changes in a dementia patient) can escalate into an emergency before anyone notices. The lack of real-time insights from the home environment makes it challenging to deliver truly preventive care. Consequently, conditions that could be managed at home can deteriorate, leading to ER visits or hospitalization that proactive intervention might have avoided.
These core problems – too few caregivers, too many avoidable hospital trips, and a reactive care paradigm – underscore the need for smarter solutions. Home health providers must find ways to do more with less, ensuring patients get timely, effective care despite resource constraints. This is where AI in home health enters the picture as a game-changer.
The Role of Predictive AI in Home Health Care
Predictive AI refers to artificial intelligence systems (often leveraging machine learning) that analyze vast health data to forecast future risks or events. In home health, these AI tools continuously monitor patient data and vital signs in real time and then alert providers to potential issues before they worsen. This marks a major shift from traditional telehealth or remote monitoring, which might simply raise an alarm when a metric crosses a threshold. Predictive AI goes further by spotting subtle trends and patterns that humans might miss:
- Rich Data Sources: Predictive models ingest data from a variety of sources – electronic health records (EHRs), caregiver notes, medication adherence logs, and remote patient monitoring (RPM) devices. Wearable sensors can track seniors’ activity levels, heart rate, blood pressure, oxygen saturation, sleep quality, and even gait or balance. Some platforms also integrate environmental data (e.g., home temperature or motion sensors) and patient-reported symptoms. By fusing these data streams, AI creates a 24/7 holistic picture of the patient’s condition.
- Proactive, Preventative Insights: Unlike manual monitoring that might trigger a response only after a fall or acute symptom, predictive AI algorithms identify risk factors early. For instance, an AI might detect that an elderly patient’s walking speed has subtly declined over a week and their nighttime bathroom visits have increased – patterns that correlate with heightened fall risk or a possible infection. The system can alert the care team to check in or adjust the care plan before an injury or ER visit occurs. This enables truly preventative health care with AI, shifting from today’s reactive model to proactive intervention.
- Machine Learning vs. Traditional Rules: Traditional RPM often relies on fixed thresholds (e.g., blood pressure > 150 triggers an alert). Predictive AI uses advanced machine learning models that continually learn an individual’s baseline and trend. It flags anomalies specific to that patient, leading to fewer false alarms and more meaningful alerts. Moreover, AI can combine multiple variables – for example, noticing a pattern of slight weight gain, increased blood pressure, and reduced physical activity that together indicate an elevated risk of heart failure exacerbation. This multidimensional analysis is far beyond what a single vital-sign threshold alert could achieve.
In short, predictive AI acts as an intelligent guardian in the background: analyzing data in real time, predicting problems days or weeks in advance, and prompting caregivers with actionable insights. By leveraging these tools, home health providers can deliver remote patient monitoring AI that not only tracks patients but actively “thinks ahead” to keep them safe. The result is more preventative, personalized care – a stark difference from the traditional one-size-fits-all visit schedule or emergency call button. Next, let’s look at some real-world applications proving the impact of this approach.
Real-World AI Use Cases in Home Health Care

Predictive AI isn’t just theoretical; it’s already driving impressive outcomes in home-based care. Here are three real-world AI use cases in home health care – from early risk detection to hospital-at-home programs – and the results they are achieving:
- CarePredict – Preventing Falls and Health Declines: CarePredict is an AI-powered platform using wearable devices to learn an elderly individual’s daily activity patterns. By tracking metrics like movement, eating, and sleep, it can detect subtle changes that precede health declines. In a peer-reviewed study, senior living communities using CarePredict saw 40% fewer hospitalizations and 69% lower fall rates compared to those without the technology. Residents also stayed 67% longer on average, as early interventions kept them healthier and out of the hospital. This use case shows how AI-driven monitoring of activities of daily living can spot risks (like emerging mobility issues or urinary tract infections) early on. Caregivers receive alerts on their mobile devices if something is amiss – for example, if a normally active client hasn’t left their bedroom by a certain time or is visiting the bathroom far more frequently than usual. Proactive care adjustments (such as hydration prompts or medication changes) can then be made to prevent a serious incident. The CarePredict example highlights how predictive AI can dramatically reduce falls and acute events among older adults at home.
- Biofourmis – Hospital at Home & Chronic Care Management: Biofourmis offers an AI-based remote monitoring solution used by health systems to manage high-risk patients at home, including those with chronic conditions or recently discharged from the hospital. Their analytics platform continuously analyzes vitals and symptoms to predict deteriorations. According to Biofourmis, deployments have achieved up to a 70% reduction in 30-day hospital readmissions for home-monitored patients. The AI can detect signs of decline 21 hours sooner than traditional approaches by identifying subtle physiologic changes. For example, in heart failure care, the system might predict an impending exacerbation and prompt a medication adjustment or telehealth visit, avoiding a trip to the ER. In addition to clinical gains, this approach has cut the cost of care by as much as 38% in some programs. Biofourmis showcases how AI-driven “virtual wards” or hospital-at-home programs can safely care for acute and chronic patients at home, intervening early to prevent readmissions. It’s a powerful validation that predictive analytics can deliver hospital-level outcomes in a home setting.
- Current Health – Transitional Care and Post-Surgery Monitoring: Current Health, a care-at-home platform (now part of Best Buy Health), combines continuous wearable monitoring with AI analytics to support patients after hospital discharge. A notable use case is with heart failure patients: By closely tracking each individual’s vitals and adherence and following up with nurse support, one health system using Current Health brought 90-day readmissions down to 0% for high-risk heart failure patients. This is a stunning result in a population that typically sees frequent rehospitalizations. The platform also helped UMass Memorial Health save over 6,000 hospital bed days in one year by shifting care to the home. These outcomes were achieved by predicting which patients were at risk of complications and intervening immediately, for instance, adjusting a care plan if a post-surgical patient’s blood pressure and activity levels indicate possible infection. Current Health’s example underlines how AI-powered transitional care can improve recovery and prevent setbacks once a patient leaves the hospital. By extending the care continuum into the home with smart monitoring, providers ensure patients don’t “fall through the cracks” in the critical days and weeks post-discharge.
Each of these use cases – fall prevention, chronic care management, and post-acute monitoring – demonstrates concrete benefits of predictive AI in home health. Fewer emergencies, fewer readmissions, and better patient stability at home translate to cost savings and improved satisfaction for all involved. Importantly, these are not isolated pilots but scalable solutions already being adopted in the industry. Next, we’ll discuss how these benefits manifest for different stakeholders in home health care.
Benefits of Predictive AI by Stakeholder
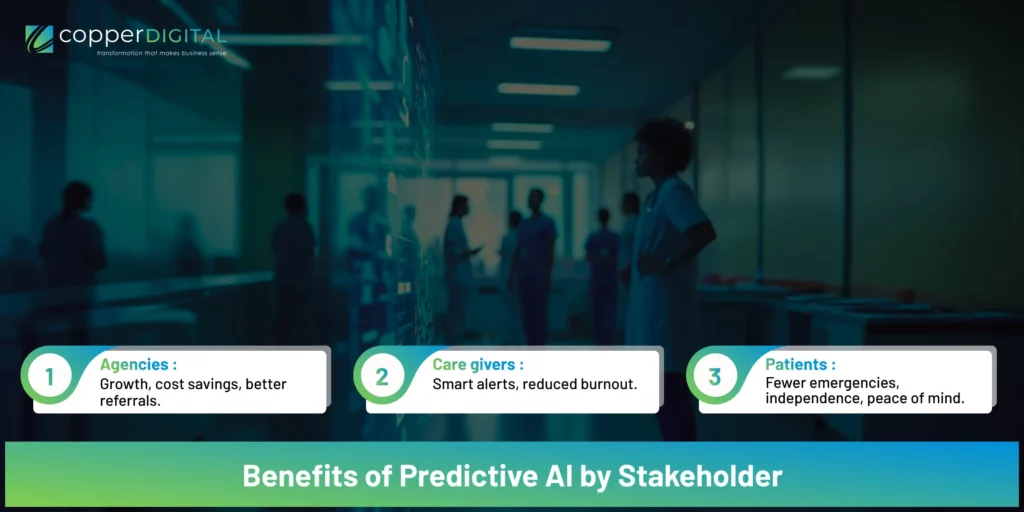
Implementing predictive AI and AI in home health monitoring delivers a range of benefits across the board – for agency owners and executives, frontline caregivers, and the patients and families receiving care. Here’s how each group stands to gain:
- For Home Health Business Owners and Administrators: Embracing home health efficiency with AI can significantly improve operational and financial performance. Agencies can handle larger patient loads with the same or fewer staff by relying on AI to prioritize which patients need attention first each day. This means higher productivity and the ability to grow services despite the labor shortage. Preventing avoidable hospitalizations and ER visits not only improves patient satisfaction but also benefits agencies in value-based payment models – better outcomes can lead to higher reimbursement and referral rates. Additionally, AI insights help in optimizing scheduling and resource allocation (for example, sending a nurse only when data indicates a genuine need, reducing unnecessary visits). Over time, these efficiency gains can lower costs and increase profit margins. Researchers estimate that broader AI adoption could save 5–10% of U.S. healthcare spending (~$200–$360 billion annually) by improving efficiency and outcomes. Home health agencies that leverage AI will be well-positioned to capture their share of these savings and outperform competitors still using manual processes.
- For Caregivers, Nurses, and Clinical Teams: Predictive AI acts like a smart assistant, triaging information and automating routine monitoring tasks. This reduces the burden on staff who would otherwise spend hours doing check-in calls or poring over data. Caregivers get real-time alerts and prioritized to-do lists, so they know exactly which patients need urgent outreach on a given day (e.g., an alert that Mrs. Smith’s blood sugar is trending upward signals it’s time to intervene). By catching issues early, AI tools help avoid crises that are stressful for staff – for instance, preventing a nighttime emergency visit through an afternoon adjustment is a win for the care team, too. Over time, this makes the job more rewarding: nurses and aides can focus on providing care and education rather than chasing problems. Importantly, by reducing burnout and moral distress, agencies may improve staff retention. As one major study noted, AI can improve day-to-day workflow for clinicians and “let them spend more time looking after patients,” thereby boosting staff morale and retention. In short, predictive AI enables caregivers to work smarter, not harder, and to deliver higher-quality care with confidence.
- For Patients and Families: This is where the impact truly shines. Patients monitored by AI benefit from greater safety and peace of mind. They are far less likely to experience medical crises at home because warning signs are caught early. This means fewer hospital trips, fewer complications, and better health outcomes. For example, a senior with COPD might avoid a dangerous flare-up because the AI flagged decreased oxygen levels and prompted a same-day medication change. Family members, too, gain reassurance knowing that their loved one is being watched over continuously by intelligent systems and a care team that will be notified at the first sign of trouble. Predictive AI also promotes independence – patients can remain in their homes longer, knowing support will proactively come to them when needed. According to the World Health Organization, the global population of people over 60 is growing at an unprecedented pace (from 1 billion in 2019 to 1.4 billion by 2030). Without innovations like AI, many of these older adults would face institutionalization or health crises. With AI, they can age in place safely while their families stay informed through apps and alerts. In surveys, patients using AI-driven virtual health assistants even report 20% better medication adherence, since reminders and engagement are tailored to them. All told, the patient experience becomes more personalized and proactive, translating to higher satisfaction and quality of life.
By delivering value to each stakeholder – efficiency and revenue upside for owners, workflow relief for clinicians, and improved outcomes for patients – predictive AI creates a win-win-win scenario in home health. Of course, realizing these benefits requires proper implementation, which we address next.
Implementation Roadmap for AI-Powered Home Health
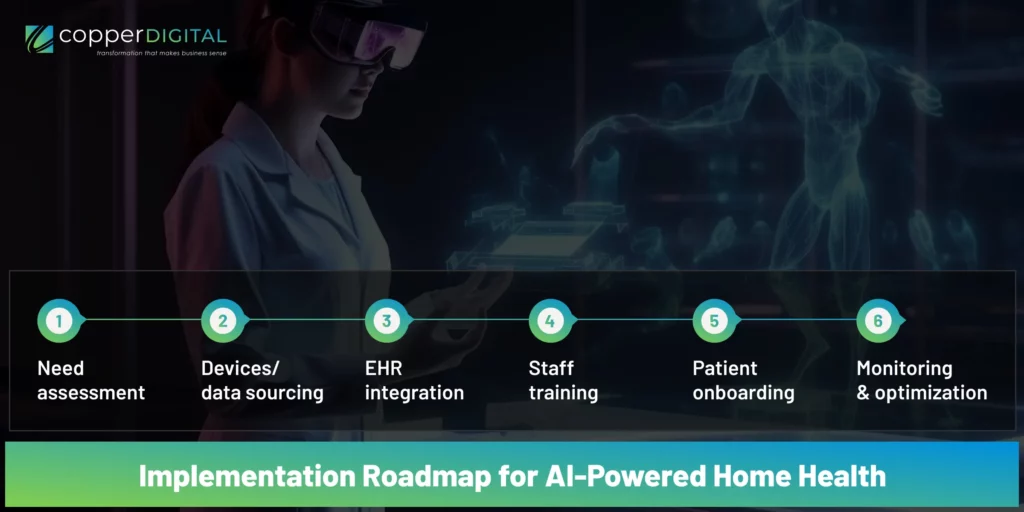
Adopting AI in home health care is a strategic process. Business owners and tech decision-makers should approach it with a clear roadmap to ensure a smooth integration of predictive tools into daily operations. Below is a step-by-step implementation plan:
- Assess Needs and Define Use Cases: Begin by identifying the key pain points or goals for your home health organization. Are you trying to reduce 30-day readmissions for post-acute patients? Improve fall prevention for your elderly clients? Or optimize nurse scheduling? Defining clear objectives will guide which AI solution or predictive models to deploy. Engage clinicians in this discovery – their frontline insights will highlight where AI can make the biggest difference.
- Choose Devices and Data Sources: Based on the use cases, select the appropriate remote monitoring devices and data streams. This could include wearable health trackers (for vitals like heart rate, blood pressure, and oxygen), in-home sensors (fall detectors, motion sensors, smart pill dispensers), and integrations with existing tools (like Bluetooth glucometers or weight scales). Ensure the devices are user-friendly for patients. For example, if targeting frail seniors, a simple wristband or passive sensor (that doesn’t require constant charging or interaction) may work best. The goal is to capture accurate, continuous data with minimal burden on the patient or caregiver.
- Integrate with EHR and Platforms: A critical technical step is integrating the AI platform with your electronic health record system or care management software. Predictive insights are most useful when they appear in the workflows your staff already uses. Work with the vendor’s tech team or your IT department to set up secure data exchange between RPM devices, the AI analytics cloud, and your EHR. This may involve HL7/FHIR interfaces or APIs provided by the AI solution. Also, establish alert delivery mechanisms – whether that’s within the EHR, a standalone dashboard, or pushing notifications to clinicians’ mobile devices. Data privacy and HIPAA compliance must be ensured at this stage; all patient data should be encrypted and access-controlled.
- Train Staff and Refine Protocols: Even the best AI is only effective if caregivers respond appropriately. Conduct comprehensive training for your nurses, aides, and care coordinators on the new AI system. They should understand what the alerts and risk scores mean, and how to take action (e.g., which threshold of risk warrants a phone call vs. a visit). Develop updated care protocols incorporating AI – for instance, “if fall risk alert is high, perform a home safety check within 24 hours” or “if AI predicts hospitalization risk >50%, escalate to NP for possible medication review.” It’s helpful to start with a pilot group of patients and a small team, gather feedback, and refine the alert parameters to minimize false positives. This phase is about change management as much as technology – reassure staff that the AI is there to support, not replace, their judgment, and encourage them to trust but verify the recommendations as they grow comfortable.
- Onboard Patients and Families: Introduce the technology to patients and their families in a positive, patient-centric way. Emphasize that these wearables or sensors will augment their care and keep them safer at home. Provide easy-to-understand instructions for any devices the patient needs to interact with, and ensure consent and understanding of data use. Some patients may worry about privacy or feel uneasy being “watched” by AI – address these concerns by explaining the safeguards and the benefits (like how it can prevent a hospital trip). Demonstrate how alerts work and possibly give family members access to a companion app or portal so they feel involved. Successful onboarding will lead to higher adherence (wearing the device, charging it, etc.) and better data for your AI to work with.
- Monitor, Measure, and Overcome Barriers: Once deployed, continuously monitor the program’s impact. Track metrics like readmission rates, fall incidents, emergency visits, patient satisfaction, and staff response times before vs. after AI implementation. These will help prove the ROI of the initiative. Be prepared to encounter some barriers: technical glitches, staff skepticism, patient dropout, etc. Have a support plan with the AI vendor to troubleshoot device issues or data inaccuracies quickly. Address workflow concerns by holding regular debriefs with your team – for example, if nurses feel there are too many alerts, work with the vendor to adjust sensitivity. Also, navigate regulatory and reimbursement aspects: document how AI-driven interventions are recorded and billed, ensuring you make use of any Medicare remote monitoring codes or value-based incentive programs. By proactively identifying challenges (like connectivity issues in rural areas or training needs for new staff) and addressing them, you’ll steadily move towards full integration.
Implementing predictive AI is not a plug-and-play overnight switch; it’s a journey of gradual adoption. But with this roadmap, agencies can start small, learn, and scale up, resulting in a robust AI-enabled home health operation. The investment in training and systems pays off when your organization begins seeing fewer crises and more streamlined care delivery. Now, let’s explore what the future holds for AI in home health and why getting on board sooner rather than later is wise.
Future Outlook and Trends in AI-Powered Home Health
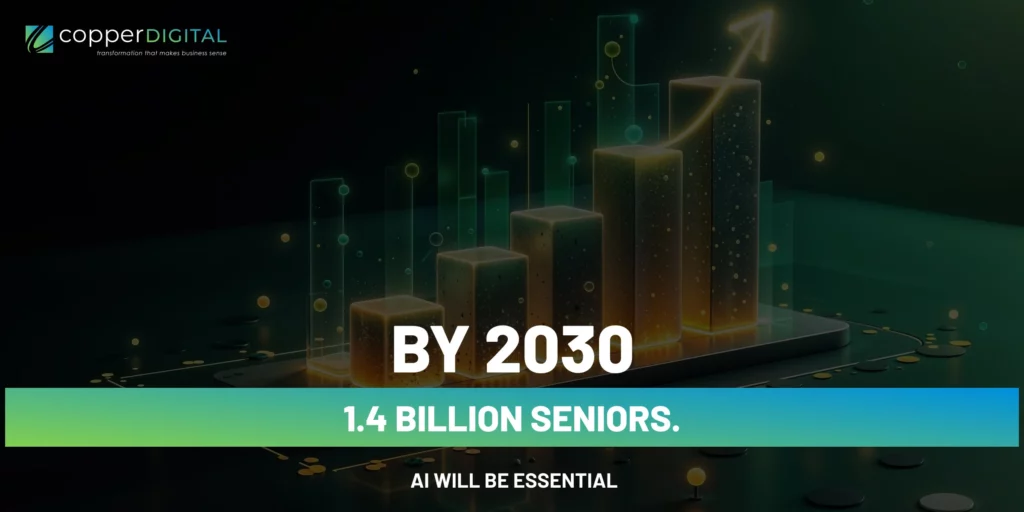
The landscape of home health care is poised for significant evolution in the coming years, and predictive AI will play a central role. Here are key future trends and considerations:
- Rising Demand from an Aging Population: By 2030, all Baby Boomers will be over 65, and older Americans will make up about 20% of the U.S. population. Globally, the number of people aged 60+ will hit 1.4 billion. This silver tsunami will intensify the strain on home health services and exacerbate workforce shortages. AI is not just a nice-to-have – it will become essential to care for so many seniors with limited human resources. International health experts note that AI will be critical in building a care infrastructure for the aging population and in combating clinician shortages and burnout. In other words, not adopting AI carries the risk of being overwhelmed by demand. Providers that fail to automate and predict will struggle to meet care needs, potentially facing higher costs and lower quality outcomes.
- Supportive Policy and Payment Environment: Policymakers are increasingly recognizing the value of remote patient monitoring, AI, and home-based care. Medicare has introduced reimbursement codes for remote monitoring and is experimenting with programs like Hospital-at-Home. The Centers for Medicare & Medicaid Services (CMS) has stated that treatment for over 60 acute conditions (like pneumonia, heart failure, COPD) can be safely managed in home settings with proper monitoring. We can expect more policy support for home health innovation, including incentive models that reward prevention over reactive care. Value-based care contracts already emphasize reduced readmissions and better chronic care management – precisely the areas where predictive AI shines. This trend means agencies investing in AI now will align well with future reimbursement models and potentially benefit from grants or funding aimed at digital health transformation.
- Advancing AI Models and Personalization: The AI algorithms themselves will continue to improve. Future predictive models may incorporate not just medical data but also social determinants of health (like loneliness, nutrition, and home environment) to paint a fuller picture of risk. They will likely become more personalized, using each patient’s genomics or unique health history to refine predictions, moving towards true precision medicine at home. Moreover, explainable AI is a growing focus; next-generation tools will do a better job of explaining why they issued a particular alert (e.g., pointing out which metrics or patterns led to a risk score). This transparency can increase trust and adoption by clinicians. We may also see AI triage systems that coordinate with multiple service lines – for instance, automatically scheduling a telehealth consult or dispatching a home health aide when a certain risk threshold is met. Essentially, the AI will evolve from a standalone tool to an integrated “brain” orchestrating various aspects of home care delivery.
- Integration of Generative AI and Virtual Care: Beyond predictive analytics, other AI technologies like conversational AI (think ChatGPT-like assistants) could become part of home health. Imagine AI chatbots that patients can talk to daily, reporting symptoms or asking questions, with the bot providing coaching or escalating issues to human nurses as needed. These virtual companions could help with medication reminders, mental health check-ins, or even basic triage. Combined with predictive analytics, this creates a powerful continuous care loop. The trend is toward a blended care model: the majority of routine monitoring and coaching is handled by AI and digital tools, while human clinicians focus on interventions that truly require their expertise. This maximizes reach and consistency of care. Forward-thinking home health providers are already exploring such hybrid care models to extend their services efficiently.
- Consequences of Staying Behind: Finally, it’s worth emphasizing the risk of not embracing these trends. Home health agencies that stick to traditional approaches may fall behind on outcomes and incur higher costs from avoidable hospitalizations. They could also become less attractive partners to health systems and payers that are looking for tech-enabled, data-driven providers. In contrast, those that invest in AI can differentiate themselves in the market – marketing their lower readmission rates, faster response times, and innovative care. Over time, we may see a divide where agencies leveraging predictive AI achieve superior CMS Star Ratings and patient satisfaction scores, while laggards struggle with penalties and reputational harm. In short, adopting predictive AI is not just about chasing the latest tech fad; it’s about future-proofing your home health business in a world that is undoubtedly moving toward data-guided, home-centric care.
The future of home health is one where preventative, personalized care at home becomes the norm rather than the exception. Predictive AI, along with supportive policies and evolving technology, is driving this transformation. The question for home health leaders is not “if” but “how” to implement these innovations effectively. On that note, let’s look at some expert perspectives and then how Copper Digital can assist in this journey.
Expert Insights on AI in Home Health Care
Leading health organizations and research bodies underscore the importance of AI in transforming home care:
- McKinsey & Company: The renowned consulting firm projects a significant shift of care to the home setting and major efficiency gains from AI. McKinsey estimates that 20–30% of hospital care could be delivered at home by 2025 with the help of remote monitoring and AI, especially for chronic conditions and post-acute care. They also highlight that wider AI adoption could save the U.S. healthcare system up to $360 billion a year, as mentioned earlier. In workforce terms, McKinsey’s research suggests that about 15% of current healthcare work hours could be automated by AI technologies, freeing up clinicians to focus on higher-value tasks. Their commentary reinforces that AI in home health is not just a tech upgrade – it’s a fundamental enabler for scaling care to meet rising demand.
- World Health Organization (WHO): The WHO has been vocal about the challenges of global aging and the need for tech-driven solutions. They report an expected shortfall of 10 million health workers worldwide by 2030, largely due to aging populations and increased chronic illnesses. To bridge this gap, WHO advocates for innovations like AI to support health workers. In the context of home care, WHO officials have noted that digital tools and AI can improve monitoring of long-term conditions and enable early interventions, reducing strain on healthcare services. Essentially, global health leaders view AI as key to achieving the goal of “healthy aging” – keeping people well at home and out of hospitals. The WHO’s endorsement gives credibility to investing in preventive health care with AI on a broad scale.
- National Institutes of Health (NIH) and Health Experts: In the U.S., the NIH and associated research institutions are actively exploring AI for aging in place. A 2024 commentary by an international panel of health leaders (including Harvard Medical School affiliates) published in a medical journal stated that AI will be critical for caring for an increasingly aging population and addressing clinician burnout. NIH-funded studies have also shown that predictive analytics combined with tailored interventions can improve clinical outcomes and reduce costs for older adults. For example, AI models have demonstrated over 85% accuracy in predicting falls using wearable data, which could dramatically improve fall prevention programs. The consensus among experts is that we have unprecedented amounts of health data available, and AI is the tool that can unlock insights from that data to guide better care. As one NIH-reviewed article put it, AI has the potential to “transform care delivery by improving outcomes, patient safety, and affordability” – a trifecta that aligns perfectly with home health needs.
These expert perspectives from McKinsey, WHO, NIH, and others all point toward the same conclusion: Predictive AI in home health care is a crucial innovation whose time has come. It addresses urgent problems (like workforce shortages and readmissions) and opens the door to higher-quality, more efficient care models. Home health providers would be wise to heed this collective advice and start integrating AI solutions as part of their strategic roadmap.
Copper Digital’s Capabilities and Next Steps
Adopting predictive AI can feel daunting, but you don’t have to navigate this transformation alone. Copper Digital is a leading digital transformation partner with deep expertise in healthcare and AI. We have helped organizations implement cutting-edge solutions to improve patient care and operational efficiency. Here’s how Copper Digital can support your home health business in this journey:
- Strategic AI Roadmap: Our team will work with you to assess your current challenges and goals, then formulate a tailored AI roadmap. This includes identifying high-impact use cases (e.g., reducing readmissions, optimizing scheduling), selecting the right technology stack, and outlining a phased implementation plan. We prioritize quick wins to demonstrate ROI early, while keeping a long-term vision for scalable digital transformation in home health care operations.
- End-to-End Solution Integration: Copper Digital offers end-to-end support – from sourcing and integrating remote patient monitoring AI devices, to setting up the data infrastructure, to customizing AI algorithms if needed. Whether you need to connect wearable sensors to your EHR or develop a custom dashboard for your care team, we have the technical know-how to make it seamless. Our solutions are HIPAA-compliant and designed with interoperability in mind, ensuring your predictive AI system becomes a natural extension of your existing workflows.
- Training and Change Management: We understand that technology is only as good as its adoption. Copper Digital provides comprehensive training for your staff to get comfortable with AI tools. We can run pilot programs, gather feedback, and refine the setup in collaboration with your clinicians. Our experts also help develop new care protocols and documentation processes aligned with AI-driven insights. The result is a staff that is confident and empowered to use AI in home health daily, rather than feeling threatened or confused by it.
- Ongoing Support and Optimization: After deployment, Copper Digital remains a committed partner. We monitor system performance, help interpret the data trends, and fine-tune the algorithms to minimize false alerts and maximize accuracy. As your census grows or new regulatory requirements emerge, we’ll help adjust the solution so it continues to deliver optimal value. Essentially, we act as your outsourced innovation team, keeping you at the forefront of AI advancements – from incorporating new predictive models to adding features like AI virtual assistants down the line.
Implementing predictive AI in home health care is a significant step, but with the right partner, it can be a smooth and rewarding process. Copper Digital has the healthcare domain knowledge, technical expertise, and dedication to guide you every step of the way.
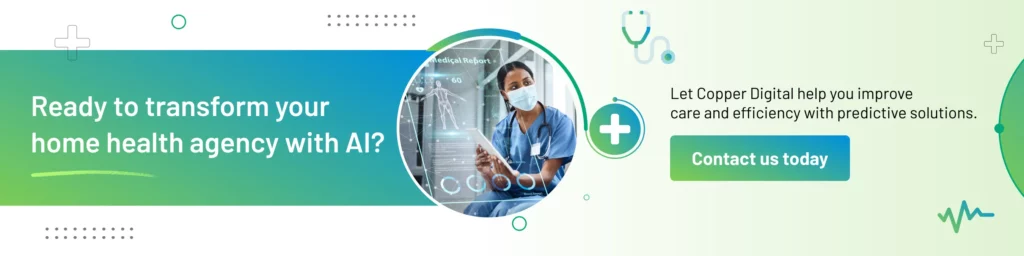
Ready to transform your home health agency with AI? Contact Copper Digital today to schedule a strategy session. We’ll discuss your unique needs and show you how our AI-driven solutions can address your staffing challenges, improve patient outcomes, and drive growth. Don’t miss the opportunity to lead in the new era of proactive, tech-enabled home care – let’s build your organization’s AI roadmap together and ensure a healthier future for your patients and your business. Get in touch with Copper Digital for a consultation and take the first step toward a smarter home health care operation.